Artificial Intelligence (AI) has witnessed stupendous growth and development over the past decades. We have seen its evolution from the simple rule-based systems to more advanced neural networks which are fully equipped with complex pattern recognition.
Despite the rapid advancements in this field, there is also a considerable struggle surrounding the AI systems in order to achieve the reasoning and understanding that is exhibited by human beings.
To bridge the gap between the two , there emerged a new concept called ‘The Neuro-Symbolic AI’, to define it precisely , It is a hybrid concept or approach that potentially aims at combining the strengths of Neural Networks and Symbolic Reasoning in order to develop more sophisticated and powerful AI systems capable of making desirable interpretations.
But before diving into the world of Neuro-Symbolic AI, let us look at the two concepts individually to have a better understanding of one of the AIBLUX’s expertise.
WHAT ARE NEURAL NETWORKS?
Neural AI is inspired from the complex and intricate design and functioning of the human brain. Neural AI incorporates artificial neural networks to learn from the input data, followed by pattern recognition and hence adapt its behavior based upon the learned experiences.
This process has achieved noticeable success in performing various experiments such as Natural Language Processing (NLP), Speech and Image Recognition to name a few.
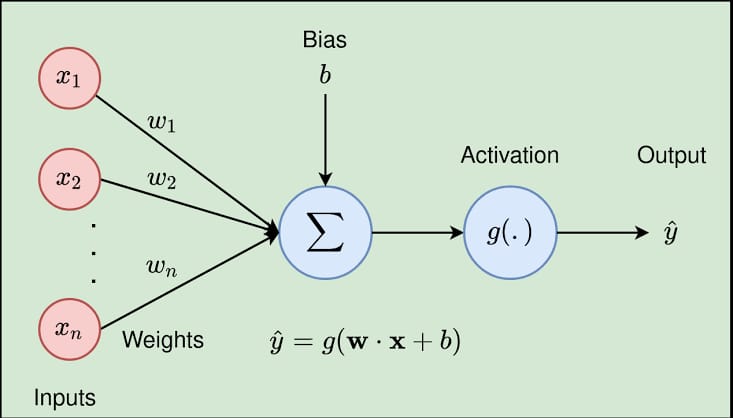
WHAT IS SYMBOLIC REASONING?
Symbolic AI draws inspiration from knowledge representation and logical reasoning. It functions by processing information via a structured and rule-based approach. This type of approach is utilized in tasks requiring symbolic manipulation and direct reasoning. It is also highly sought after in the applications in which interpretability and transparency are fundamental requirements.
It should also be noted that while both the Neural AI and Symbolic AI have achieved significant growth in their respective areas, they also pose certain limitations that may hamper their efficiency in dealing with the complicated real-world problems.
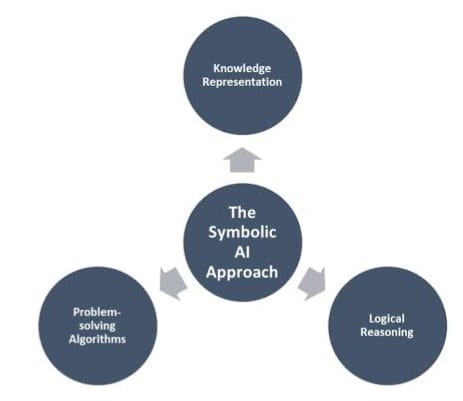
Neural AI may struggle with the challenges pertaining to logical reasoning and interpretation.
Symbolic AI could face difficulty in dealing with the uncertainty and large-scale data processing
THE ADVENT OF NEURO-SYMBOLIC AI: THE BEST OF BOTH WORLDS IN ARTIFICIAL INTELLIGENCE
The convergence of Neural Networks with Symbolic Reasoning is absolutely a Game-Changer. The neuro-symbolic paradigm provides reliable and compelling solutions to cope with these limitations
Hence, it will be appropriate to hypothesize that by combining the strengths of the learning capabilities of neural networks with the symbolic reasoning, the resultant hybrid approach of the Neural-Symbolic AI has a great potential to bridge the gap between the two, thus allowing the AI- systems to demonstrate an elaborated understanding of the complex and gigantic data sets providing more informed decisions.
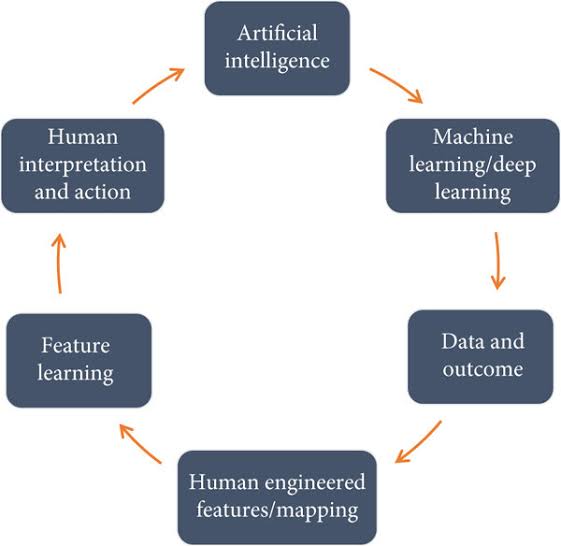
Moreover, with the ability to adapt to more dynamic and complex environments with greater efficiency and flexibility, this integrating approach will not only expand the powers of AI systems but also pave the way for new horizons of research, developments and innovations in the vast field of artificial intelligence.
MAJOR OBJECTIVES OF THE NEURO-SYMBOLIC AI:
1) To address even more complex problems involving the large data sets.
2) To learn to perform various tasks at a time rather than the individual and specific task.
3) To adopt the judgements as well as the behaviors that are both reasonable and can be comprehended.
THE KEY COMPONENTS OF NEURAL-SYMBOLIC AI: AN OVERVIEW OF THE TRAINING PROCESS
The Neuro-Symbolic AI working structure can be divided into three major components.
1) Neural Networks for Perception:
The main function of neural networks is to carry out the processing of the raw input sensory data. It can be in the form of images, text or audio. Through training, these networks learned to extract the relevant patterns and features from the raw unstructured data and provide the basis for the higher-level of reasoning as the output.
2) Symbolic Reasoning for Logic and Knowledge Representation:
This reasoning component is designed to handle the tasks or data that require extensive knowledge representation and logic. It utilizes the formal languages such as knowledge graphs, first-order logic to represent the rules, facts and relationships.
3) Integration Mechanisms:
Integrating both the neural networks and symbolic reasoning incorporates the processes that allow the AI systems to enable the uninterrupted collaboration and communication between the two individual components. These mechanisms include but not limited to – Hybrid Learning Algorithms, Neuro-Symbolic Architectures and symbolic embeddings.
REAL-WORLD APPLICATIONS IN VARIOUS INDUSTRIES:
1) Healthcare Setups:
In the healthcare settings, the Neuro-Symbolic AI can be helpful in improving the diagnostic systems by integrating the pattern recognition capabilities of neural networks with the expert reasoning and knowledge powers of the symbolic AI. Hence, it will improve the efficiency and accuracy of the diagnosis in the field of medicine.
2) Drug Discovery:
It is also helpful for the researchers and in the pharmaceutical industry to speed up the drug discovery process. It can do so by assisting in the identification of the suitable candidates by thorough analysis of the chemical structures and the biological pathways.
3) Knowledge Graphs and Ontologies:
This AI system can optimize the knowledge graphs and ontologies to reason and represent the complex relationships in the data. In this way, it will be helpful to perform the valuable tasks such as extracting the knowledge, semantic research and effective retrieval of the useful information.
4) Robotics and Autonomous System:
In the domains of robotics and autonomous vehicles, neuro-symbolic AI can help in improving the decision making by incorporating the perception from the neural-networks by using the sophisticated and high-level planning and reasoning from the symbolic AI systems.
5) Natural Language Processing (NLP):
It can also be helpful in expanding the understanding and generation of the natural language which can provide more sophisticated language models and dialogue systems.
6) Financial Fraud Detection:
Neuro-Symbolic AI is also helping financial institutions and banks to identify and catch the fraudsters. Traditional AI might notice something fishy in your transactions patterns, but neuro-symbolic AI is many steps ahead. It can apply the banking regulations to figure out if those patterns are suspicious or not and can also cross-check them against the known fraud indicators and legal standards. It can also be helpful in risk assessment and designing the investment strategies within the finance sector.
7) Education and Tutoring Sector:
Neuro-Symbolic AI can help in the development of the education sector by providing personalized education after understanding the behaviour of the students via neural networks and generating the tailored teaching methods via the symbolic reasoning.
8) Others
In short, the Neuro-Symbolic AI system can be incorporated with any domain across the globe to provide ease in the lives of the consumers and businesses. Some other areas of where it can be efficiently used to level up the game include Supply Chain Optimization, Retail Industry, Risk Management, Customer Support, Cybersecurity, Environmental Monitoring.
LATEST TRENDS AND STATS IN NEURO-SYMBOLIC AI: DECODING THE LITERATURE
The interest in the Neuro-Symbolic AI hybrid approach has been growing day by day. According to the data presented by Allied Market Research, the AI market will be witnessing a growth trajectory from $ 387.45 billion in 2022 to $1.39 trillion by 2029 primarily due to the innovations in Neuro-Symbolic AI.
IBM and MIT, the key industry players are investing heavily in neuro -symbolic AI research. Project Debater by IBM is designed to utilize the combination of neural networks and symbolic AI so that it can understand the human language at a much deeper level.
In another report by Markets and Markets, by 2025 the hybrid AI systems including the neuro-symbolic AI will be able to drive the 45% of enterprise AI solutions in various sectors like healthcare, finance and transportation.
CHALLENGES AND ETHICAL CONSIDERATIONS IN SCALING THE NEURO-SYMBOLIC AI:
AI systems like the one under discussion also pose certain challenges that could stunt technological advancements. Here is the brief overview of the challenges that may arise in up-scaling the Neuro-Symbolic AI.
1) Scalability:
Handling the large data sets and complex problems is a havoc, hence it is important to ensure that neuro-symbolic AI systems are able to handle the huge datasets.
2) Complex Integration:
It is crucial to carefully design and optimize the integration of neural networks with symbolic reasoning systems as both are complex and have their own individual limitations
3) Resource Demands:
Due to the hybrid nature, the neuro-symbolic AI systems often need hue computational resources which can be considered as a significant hindrance in its widespread adoption.
4) Training and Optimization:
Another significant challenge is the development of effective training methodologies that could balance the learning abilities of neural networks with the requirements and limitations of the symbolic reasoning.
Furthermore, to ensure the fairness and unbiased decision-making process, it is important to address the ethical considerations such as bias in knowledge, reasoning and representation. With, ensuring and promoting the explainability and transparency is fundamental in building accountability and trust in AI technologies.
THE FUTURE OF AI: HOW NEURAL-SYMBOLIC AI WILL SHAPE A BETTER TOMORROW
The Neuro-system AI presents a bright future. The road to its exponential growth lies in enhancing the reasoning mechanisms, refining knowledge representations, improving the system scalability and efficiency as well as prioritizing the ethical considerations. In this regard here are the few suggestions
1) Addressing Bias:
It can be done by combining the data sources and enforcing the required regulations.
2) AI and Human Collaboration:
AI systems will increase the decision-making by providing the recommendations according to data and logic hence improving the collaboration between the AI and human.
3) Connecting to the Internet of Things (IoT):
IoT generates enormous data. Neuro-symbolic AI can do so. Empower the devices to become more intelligent and autonomous by interpreting the data.
4) Enhanced Transparency:
With the increasing complexity, the demand for transparency is also on the rise. It is important to ensure the transparency to provide the coherent justifications of the decisions.
TO SUM UP:
In conclusion, it can be inferred that the Neuro-symbolic AI is shaping up to be a breakthrough in using artificial intelligence systems and hence bridging the gap between the Neuro AI and the symbolic reasoning AI. It has also revealed potential avenues and promising insights for advancing the vast subject of artificial intelligence by blending the strengths of both the individual components. In this way, it also offers a novel approach to overcome the shortcomings of the traditional AI paradigms.
CALL TO ACTION:
If you are a business looking forward to playing with Neuro-symbolic AI systems in your prospective projects, then worry not and let us take the front seat and witness a wonderful journey of continued exploration and innovation. Together, we can vision the future in which the intelligent systems and models not only survive in complexity but also uphold the core values of integrity and humanity. We are looking forward to hearing from you, Contact AIBLUX.